Designing oncology drugs to hit more than one biochemical target could lead to more effective drugs, but it’s a battle getting there.
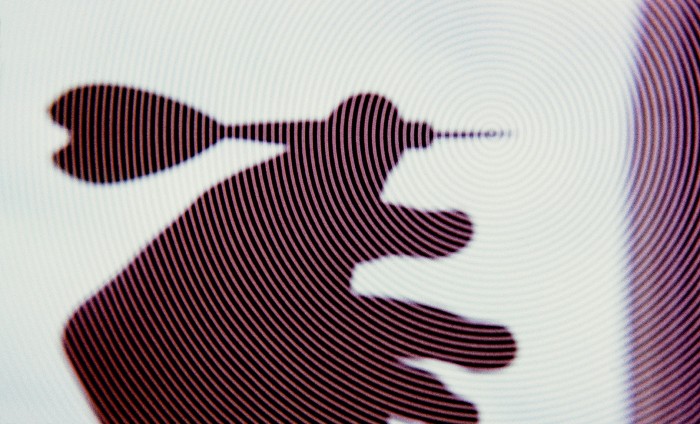
The Image Bank / Getty Images Plus’
In May, the Canadian company Cyclica unveiled a new software platform at the Collision conference in Toronto that it hopes will help usher in medicines that are more effective while preventing unwanted side effects. Its product, a platform known as Ligand Design, comes up with new drug constructs that interact with multiple specific targets, such as those on the surface of tumor cells. The creators of the tool say it harnesses the multifaceted behavior of drugs that scientists often ignore. “It has been validated that drugs do more than one thing, but classical design has been focused on figuring out the best design for only one target,” says Naheed Kurji, president and CEO at Cyclica.
This new tool is part of an approach called polypharmacology, which gained attention in 2005 thanks to the work of organic chemist Zoran Rankovic — then at the UK company Organon and now at St. Jude Children’s Research Hospital in Memphis, Tennessee.
Experts agree that a multitargeted approach could create new medicines that work better and cause fewer bad side effects because a useful method looks for effective targets to hit and toxic ones to miss. Scientists at Toronto-based Tieös Pharmaceuticals used Ligand Design to search for new cancer drugs, and cofounder Arun Anand says, “The molecules we’ve synthesized have shown good solubility, multitargeted biophysical interaction and have evidenced good safety, efficacy and pharmacokinetics in our in vivo models.” He adds that his team has “been blown away by what we are seeing.”
With adequate target coverage, an increase in computational power through cloud computing and algorithmic advances, the time could be right for an integrated polypharmacological approach to drug discovery. Heather Carlson, an expert in bioinformatics and structure-based drug design at the University of Michigan College of Pharmacy, says, “This is a great time to tackle polypharmacology, specifically because informatics tools are readily available to the public.” Big pharmaceutical companies also have projects in the mix, especially for fighting cancer.
Crunching the computation
Cyclica took five years to analyze information about biological structures, such as targets, and potential small-molecule drugs with a proprietary neural-net algorithm. In contrast with traditional approaches that screen libraries of drug compounds against a single target, Ligand Design generates new molecule structures across multiple targets in a computer simulation. “This platform can explore a much larger chemical space — trillions of times larger than any library of existing drugs, within the context of the whole proteome — at practically no cost and within one day,” Kurji says.
Given the recent release of Ligand Design, more scientists have used similar, but older, computational tools from Cyclica, such as Ligand Express, which uncovers polypharmacology by screening existing small molecules to see which ones could bind multiple protein targets. For example, Friedrich Rippmann, director of computational chemistry and biology at Merck KGaA, Darmstadt, Germany, says Ligand Design’s “technology fits well into our increasing focus on phenotypic high-throughput screening, adding a target deconvolution method that takes 3D target information into account, which is a unique feature.”
Polypharmacology is not all about searching the unknown. Instead, scientists can work with known cancer-related pathways and make a molecule that impacts more than one of them. For example, in February, Merck KGaA, Darmstadt, Germany and UK-based GlaxoSmithKline announced an alliance to develop a bifunctional fusion protein, bintrafusp alfa. The drug is designed to attack various cancers via two pathways. Both targets — transforming growth factor-β (TGF-β) and anti-programmed cell death ligand-1 (PD-L1) — suppress the immune system. So, blocking those targets with bintrafusp alfa could allow the immune system to attack more than one kind of cancer, including non-small-cell lung cancer, and this is being tested in a phase 2 clinical trial.
The multitargeting, though, multiplies some development challenges. “In reality, you quadruple the effort, because you have to think about reactivity and cross-reactivity,” says Joern-Peter Halle, head of the translational innovation platform at EMD Serono (part of Merck KGaA) in Massachusetts. Plus, he says that these molecules are harder to make.
Getting to know targets
One challenge for multitargeted drugs is knowing enough about the potential targets. “You need to know how those targets interact in biological systems,” says Lei Xie, a chemist and computer scientist at the City University of New York. “That requires analyzing lots of data, which takes computation.” After identifying targets, a scientist runs computational screening to find compounds that might bind that target. These steps depend on combining various forms of information — biology, chemistry and beyond — in the right way.
To do that, Xie and his colleagues developed 3D-REMAP, which combines data and information from biophysics, genomics and structural biology. Then, 3D-REMAP analyzes the data using machine learning. To validate this tool, Xie and his colleagues addressed an ongoing clinical challenge: anti-cancer drugs increasing the risk of heart failure. Instead of using the multitargeting approach to target cancer in two ways, the scientists used 3D-REMAP to find drugs that reduce the risk of heart failure and fight cancer.
As an example, 3D-REMAP predicted that levosimendan — a drug used to reduce heart-failure risk — inhibits kinases that should make this drug fight various kinds of cancer, including lymphoma1. The paper, which they published in June, also showed that the drug inhibited the growth of cancer cells in laboratory experiments.
Xie says it’s important to contrast the calculations about which structures might work best with actual, observed experimental data and to employ machine learning in the process. The observed chemical genomics can be used to train a machine model to look for the right interactions. “A fundamental problem with machine learning is that you cannot predict outside the training space,” Xie says. “So, we increase the training space by adding complementary information from structure analysis.”
(원문: 여기를 클릭하세요~)