Misleading terminology and arbitrary divisions stymie drug trials and can give false hope about the potential of tailoring drugs to individuals, warns Stephen Senn.
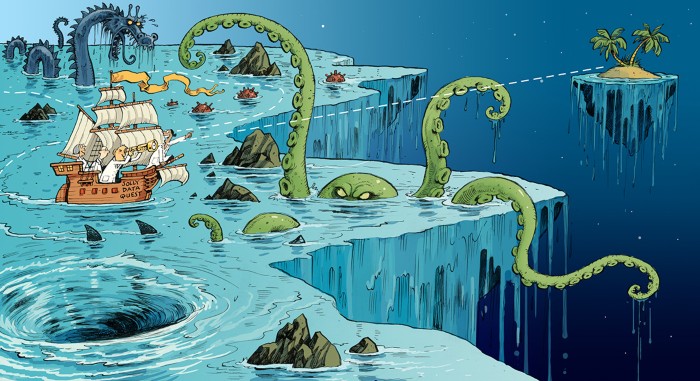
Illustration by David Parkins
Personalized medicine aims to match individuals with the therapy that is best suited to them and their condition. Advocates proclaim the potential of this approach to improve treatment outcomes by pointing to statistics about how most drugs — for conditions ranging from arthritis to heartburn — do not work for most people1. That might or might not be true, but the statistics are being misinterpreted. There is no reason to think that a drug that shows itself to be marginally effective in a general population is simply in want of an appropriate subpopulation in which it will perform spectacularly.
The reasoning follows a familiar, flawed pattern. If more people receiving a drug improve compared with those who are given a placebo, then the subset of individuals who improved is believed to be somehow special. The problem is that the distinction between these ‘responders’ and ‘non-responders’ can be arbitrary and illusory.
Much effort then goes into the effort to uncover a trait to explain this differential response, without assessing whether or not such a differential exists. I think that this is one of many reasons why a large proportion of biomarkers thought to distinguish patient subgroups fall flat. Researchers need to be much more careful.
To be clear, I am not talking about research, often in cancer, that defines subpopulations of patients in advance. In that scenario, the aim is to test prospectively whether a particular drug works better (or worse) in people whose cancer cells have a specific genetic defect — a biomarker such as a HER2 mutation in breast cancer or the BCR–ABL fusion gene in leukaemia. (It’s worth stating that the overall percentage of US patients with advanced or metastatic cancer who benefit from such ‘genome-informed’ cancer drugs is estimated to be less than 7% at best2; the proportion is likely to be lower for those whose cancer is at an earlier stage.)
What I take issue with is the de facto assumption — often made in studies of chronic diseases such as migraine and asthma — that the differential response to a drug is consistent for each individual, predictable and based on some stable property, such as a yet-to-be-discovered genetic variant.
S. Senn
Consider an actual clinical trial in which 71 patients were treated with two doses. Twenty ‘responded’ to both doses, 29 to neither dose and 14 to the higher dose, but not the lower one. That is as expected. More surprising is that eight ‘responded’ to the lower dose and not the higher one, which is at odds with how drugs are known to work. The most likely explanation is that the ‘response’ is not a permanent characteristic of a person receiving the treatment; rather, it varies from occasion to occasion. In this example, the fact that two doses of the same drug were being compared alerts us to the need to consider that source of variability. If the comparison instead involved different molecules, researchers might then overlook the explanation of occasion-to-occasion variation and jump to the conclusion that the results must reflect a differential response.
I have seen unsubstantiated interpretations waft through the literature. They start with trials designed to show whether a drug works, and then get misinterpreted. For example, a 2005 study3 found that one ulcer treatment led to healing in 96% of patients after 8 weeks, and another treatment healed 92% of patients, a difference of 4%. This finding filtered into a 2006 meta-analysis4, and then a third article1 followed an all-too-common statistical practice, stating that only 1 in 25 (or 4%) of patients would benefit from the first ulcer treatment. It is not hard to imagine other researchers carrying out futile work to try to understand why.
Trial traps
Here are some common pitfalls.
Lazy language. Participants in clinical trials are often categorized as being responders or non-responders on the basis of an arbitrary measure of improvement — such as a certain percentage drop in established clinical scales that assess depression or schizophrenia. It does not necessarily follow that any individual who improves owes that improvement to the treatment. Researchers who acknowledge in the methods section of a paper that an observed change is not a proven effect of a drug often forget to make that distinction in the discussion. Variations are uncritically attributed to characteristics of the person receiving treatment rather than to numerous other possibilities.
Arbitrary dichotomies. Other classifications can depend on whether a participant falls on one side or another of a boundary on a continuous measurement. For example, a person with multiple sclerosis who relapsed 364 days after treatment is a non-responder; one who relapses 365 days after treatment is a responder. This is simplistic — it recasts differences of degree as differences of kind. Worse, it causes an unfortunate loss of information, and means that clinical trials must enrol more participants than would otherwise be needed to reach a sound conclusion5,6.
Participants’ variability. Physiology fluctuates. Trial participants are often labelled as responders after one measurement, post-treatment, with the tacit assumption that the same treatment in the same person on another occasion would yield the same observation. But repeated observations of the same person with a disease such as asthma or high blood pressure show that the result after treatment can vary.
Inappropriate yardsticks. Judging whether a drug works depends on making assumptions about what would have happened without the treatment — a counterfactual. One common technique for estimating the counterfactual is to take baseline measurements; for instance, the volume of air that people with asthma can force from their lungs in one second at the start of a trial. But baselines are a poor choice of counterfactual. Guidelines agreed by drug regulators in the European Union, Japan and the United States disparage their use as controls.
There are many reasons besides treatment — such as regression to the mean or variation in clinical settings — that might explain a difference from baseline, especially if measurements such as elevated blood pressure or reduced lung capacity are used to determine who can enrol in a clinical trial. Let’s say Patient X was enrolled in a trial after meeting the criteria for having a blood-pressure measurement of more than 130/90 mm Hg. She is given a drug, after which her blood pressure measures 120/80 mm Hg. One possibility is that the drug affected her blood pressure. Another is that 125/85 mm Hg (or some other intermediate value) is her mean blood pressure, and that she had a bad day on enrolment and a good day later. Yet another possibility is that her blood pressure was measured at different times of the day, at different places or by different people.
For measurements such as pain scores and cholesterol levels, predictions for individuals — based on an average of all participants — can be more accurate than predictions based on an individual’s own data taken just once7.
Rates of response. Suppose that in a large trial for an antidepressant, 30% of patients have a satisfactory outcome in terms of their score on the Hamilton Depression Rating Scale after taking a placebo, and 50% show a satisfactory outcome after taking the drug. This means that the probability of a good outcome observed with the drug is 20% higher than with the placebo. Or put another way, on average, if five patients were treated with the drug, one more would experience a satisfactory outcome. This statistic is an example of what is called the ‘number needed to treat’ (NNT).
This concept was introduced 30 years ago8 and is extremely popular in evidence-based medicine and assessments of health technology. Unfortunately, NNTs are often falsely interpreted. Consider a trial comparing paracetamol to a placebo for treating tension headache. After 2 hours, 50% of people treated with the placebo are pain-free, as are 60% of those who were treated with paracetamol. The difference is 10% and the NNT is 10. However, if paracetamol works for 100% of participants in 60% of the times they are treated, it will give the same NNT as if it works for 60% of the participants 100% of the time.
A high NNT should not be taken to imply that a drug works really well for a specific, narrow subset of people. It could simply mean that a drug is just not that effective across all individuals.
Subsequence, not consequence. All of the errors discussed so far lead to the assumption that what has happened, for good or ill, has been caused by what was done before — that if a headache disappeared, it was because of the drug. It is ironic that the evidence-based-medicine movement, which has done so much to enthrone the randomized clinical trial as a principled and cautious way of establishing causation across populations, consistently fails to establish causation in the context of personalized medicine.
Way forward
These warnings are not intended to discourage researchers from pursuing precision medicine. Rather, they are meant to encourage them to get a better sense of its potential at the outset.
How to improve? One thing we need more of are N-of-1 trials. These studies repeatedly test multiple treatments in the same person, including the same treatment multiple times (see ‘Compare each patient at least twice’).
With such designs, we can assess differences between the same drug being administered on many occasions, and compare those data with differences seen when different drugs are administered in the same way. They are being used, for example, in trials of fentanyl for pain control in individuals with cancer9 and of temazepam for people with sleep disturbances10.
When medicines are given on many occasions for a chronic or recurring condition, N-of-1 studies are a good way of establishing the scope for personalized medicine11. When drugs are given once or infrequently for degenerative or fatal conditions, careful modelling of repeated measures can help. Whatever their approach, trial designers must hunt down sources of variation. To work out how much of the change observed is due to variability within individuals requires more careful design and analysis12.
Another advance would be to drop the use of dichotomies5. Statistical analysis of continuous measurements is straightforward but underused. More-widespread uptake of this approach would mean that clinical trials could enrol fewer patients and still collect more information6.
Perhaps the most straightforward adjustment would be to avoid labels such as ‘responder’ that encourage researchers to put trial participants in arbitrary categories. An alternative term — perhaps ‘clinical improvement’ or ‘satisfactory endpoint’ — might help. Better still, sticking with the actual measurement would reduce the peril of all the pitfalls mentioned here.
It has been a long, hard struggle in medicine to convince researchers, regulators and patients that causality is hard to study and difficult to prove. We are in danger of forgetting at the level of the individual what we have learnt at the level of the population. Realizing that the scope for personalized medicine might be smaller than we have assumed over the past 20 years will help us to concentrate our resources more carefully. Ironically, this could also help us to achieve our goals.
Nature 563, 619-621 (2018)
원문: 여기를 클릭하세요~